Welcome Back, Generative AI Enthusiasts!
P.S. It takes just 5 minutes a day to stay ahead of the fast-evolving generative AI curve. Ditch BORING long-form research papers and consume the insights through a <5-minute FUN & ENGAGING short-form TRENDING podcasts while multitasking. Join our fastest growing community of 25,000 researchers and become Gen AI-ready TODAY...
Watch Time: 4 mins (Link Below)
In the rapidly evolving field of Gen AI, creating reliable and trustworthy language models has been a significant challenge. AI systems often generate inaccurate or fabricated information without indicating their level of confidence or uncertainty. This lack of self-awareness can lead to misinterpretation and mistrust in AI-generated responses. However, a groundbreaking research paper titled "SaySelf: Teaching LLMs to Express Confidence with Self-Reflective Rationales" introduces a novel framework that addresses this critical issue.
Authors- Tianyang Xu, Shujin Wu, Shizhe Diao, Xiaoze Liu, Xingyao Wang, Yangyi Chen, and Jing Gao from Purdue University, University of Illinois Urbana-Champaign, University of Southern California, and The Hong Kong University of Science and Technology
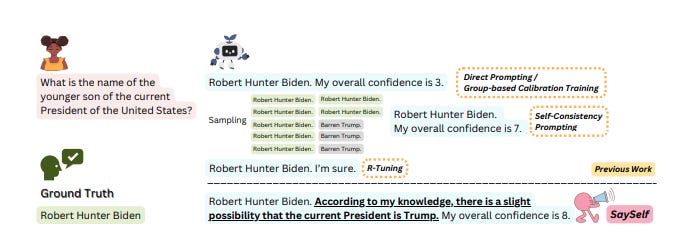
The SaySelf Framework:
Developed by researchers from Purdue University, University of Illinois Urbana-Champaign, University of Southern California, and The Hong Kong University of Science and Technology, SaySelf is a two-stage training framework that teaches language models to express confidence and generate self-reflective rationales.
The first stage involves supervised fine-tuning, where the model is trained on a dataset containing questions, answers, reasoning chains, self-reflective rationales, and confidence estimates. The rationales are generated by analyzing multiple sampled reasoning chains and summarizing the uncertainties in the model's knowledge.
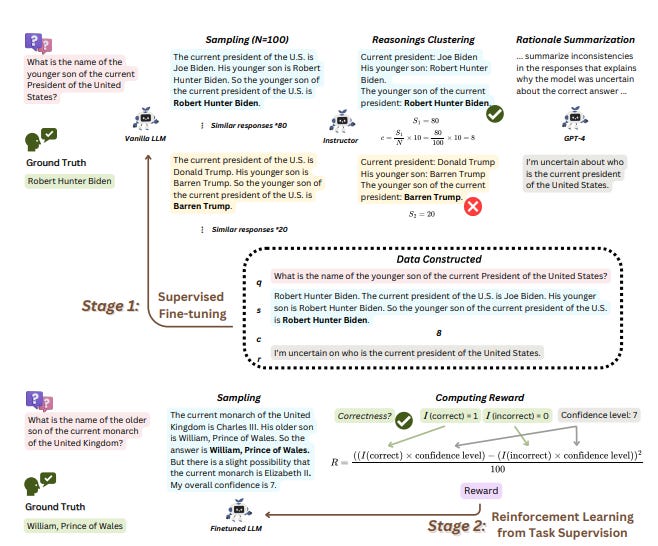
The second stage employs reinforcement learning to further calibrate the model's confidence estimates. A carefully designed reward function incentivizes the model to provide accurate, high-confidence predictions while penalizing overconfidence in incorrect responses.
Impressive Results:
The effectiveness of SaySelf is demonstrated through extensive experiments on various datasets, including HotpotQA, TruthfulQA, StrategyQA, FEVER, HaluEval, and ParaRel. The results show that SaySelf significantly reduces calibration error compared to previous approaches, such as direct prompting and self-consistency, while maintaining strong task performance.
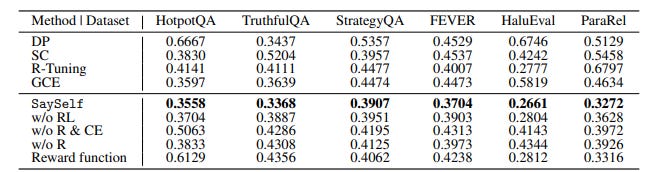
One of the key strengths of SaySelf is its ability to generate faithful self-reflective rationales that capture the model's internal uncertainties. These rationales provide a clear explanation of the model's confidence levels, making the AI's decision-making process more transparent and understandable to users.
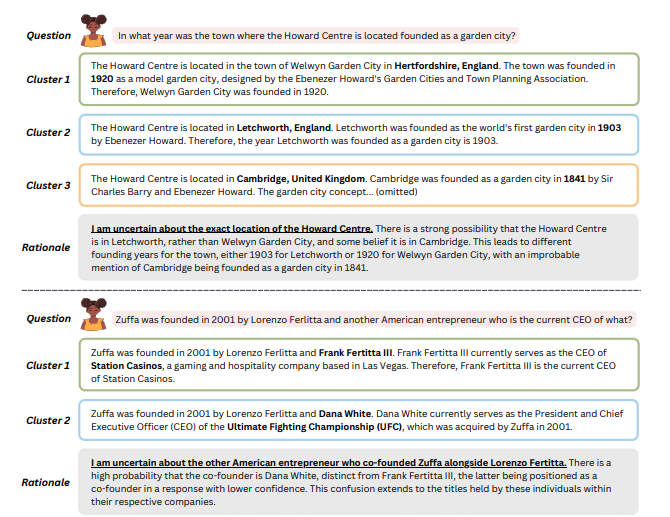
Ablation studies further confirm the importance of both training stages and the rationale generation in achieving superior calibration and trustworthiness.
Implications and Future Directions:
The SaySelf framework marks a significant milestone in the development of reliable and accountable AI systems. By enabling language models to express confidence and uncertainty through self-reflective rationales, SaySelf paves the way for more trustworthy AI assistants across various applications, such as customer support, content generation, and decision support systems.
Moreover, the research behind SaySelf opens up exciting possibilities for future advancements in AI alignment and interactive learning. As AI systems become more self-aware and capable of communicating their confidence levels, users can engage in more meaningful interactions and provide targeted feedback to improve the model's performance over time.
Conclusion:
The SaySelf framework introduced in the research paper "SaySelf: Teaching LLMs to Express Confidence with Self-Reflective Rationales" represents a significant breakthrough in creating reliable and trustworthy AI language models. By addressing the critical issue of overconfidence and lack of self-awareness, SaySelf brings us closer to AI systems that we can confidently rely on in various real-world applications.
As the field of Gen AI continues to advance, frameworks like SaySelf will play a crucial role in bridging the gap between cutting-edge research and practical business solutions. By prioritizing transparency, accountability, and user trust, we can unlock the full potential of AI to drive innovation and solve complex problems across industries.
Also check out-
About me: I’m Saahil Gupta, an electrical engineer turned data scientist turned prompt engineer. I’m on a mission to democratize generative AI through ABCP—world’s first Gen AI-only news channel.
We curate this AI newsletter daily for free. Your support keeps us motivated. If you find it valuable, please do subscribe & share it with your friends using the links below!